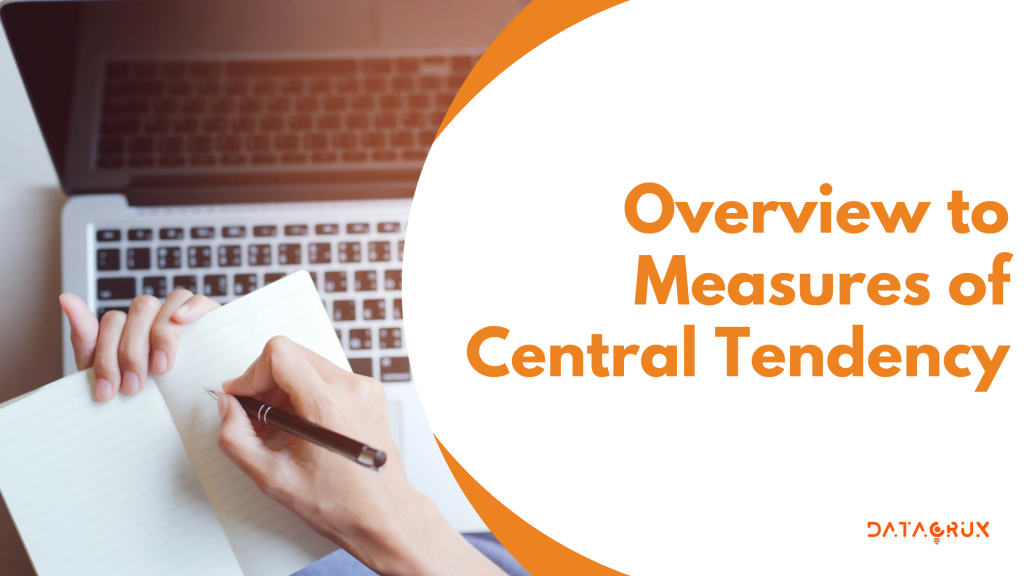
Overview of Measures of Central Tendency
Measure of central tendency
A measure of central tendency is a summary statistics that represents the center point or a typical value of a data set.
These measures indicates where most values in a distribution fall and also known as central location of distribution.
In simple way tendency of data to cluster around a middle value.
Some of the most common measure of central tendency are:
Level of Measurement and Measures of Central tendency
For nominal variables, we can only describe mode (the value that occurs the most).
For ordinal variables, we can describe the mode or median (the middle value). Median is preferred value in case of ordinal variables.
For numerical data, the mean is the preferred measure. The mean is the arithmetic average.
Uses of central tendency
- The measure of central tendency can be used as a standard for judging the relative positions of other items in the same set of data (whether a number falls above or below the average and how far away it is from the average).
- A measure of central tendency can be used to compare the relative sizes of two different data sets. Let’s say for comparing the averages of two data sets.
- It is also used to study measures of dispersion in simple words spread of data.
Link of measure of dispersion
Characteristics of Central tendency
There are certain guidelines for choosing the particular measure of central tendency.
A measure of central tendency is good if it has following properties-
- It should be easy to calculate.
- Easy to understand.
- Based on all the observations.
- Should not be affected by extreme values.
- Should be close to maximum number of observed values as possible.
Mean
The mean is the arithmetic average for calculating the mean. Mean is central value of finite set of numbers.
Let’s consider you have a data set with n values as follows
Notations:
∑ =This is the Greek letter sigma which means sum up of the numbers.
n = sample size
The mean is the most common measure of central tendency but has a huge downside as it is easily affected by outliers.
Population:
The entire set of objects or individuals or interests or the measurements obtained from all individuals or objects of interests. It can be either finite or infinite.
Sample:
A portion or part of population is known as sample.
In the similar fashion we have different formula of mean for both sample and population
Median
The median is the middle value that splits the data set into half. The method of finding median varies whether your data set has odd or even number of values.
It is a value of the variable that divides a set of data into two equal groups so that half the observations have values smaller than the median, and half the values larger than the median.
- For odd number of values sort the numbers and select the middle values.
- For even number of values sort the numbers take middle two numbers and divide by 2.
The median is the preferred measure of central tendency for ordinal variable.
The median is the measure of choice when a numerical variable has some few unusually high or low values in set of data. If this occurs mean is not a suitable measure of central tendency in majority of cases.
If a frequency distribution for ordinal data is given, the cumulative percent reports the percent of cases that fall in or below each category or a particular value.
The median is the value of the variable below which 50 % of the cases lie.
The median occurs at the value of the variable where the cumulative percent reaches its first 50 % of cases.
Always remember we cant find the median for nominal data.
Mode
The mode is the value which occurs most number of times in a data set.
Mode can also be said as the response category of a variable that is most frequently chosen by the respondents.
In the frequency distribution, the mode is the category that has the largest frequency.
When you observe any bar chart or histogram, the mode is the tallest bar among all others.
The mode is the only measure of central tendency that can be used for all levels of measurement whether it is nominal, ordinal, interval and ratio.
Also when a particular distribution has one mode we say that distribution as uni-modal. If distribution has two modes then it is known as bi-modal. In case there are several modes it is called multi-modal.
Let’s take an example
Given a data set of heights of student in a class. Find the mean, median, mode
Heights (in cm) = {180, 167, 154, 142, 181, 145, 143, 145, 167, 145}
No. of observations = 10
Mean = (180+167+154+142+181+145+143+145+167+145)/10
= 156.9 cm
So, the mean calculated is 156.9 cm
So for finding the median let’s rearrange the data in Ascending order
Rearranged heights = {142,143,145,145,145,154,167,167,180,181}
Number of observations are 10 so n is even
If the number of observations (n) is even:
Find the value of position (n/2)
So (10/2) = 5 i.e. 5th position = 145
Find the value of the position (n/2)+1
So (10/2)+1 = 5+1 = 6 i.e. 6th position = 145
Find the average of two values to get the median
Median = (145+145)/2 = 149.5
So, median is 149.5
For calculating the mode we require frequency table
Highest frequency is of 145 i.e. 3
So Mode = 145
If you observe the difference between the value of mean and median is large they are not close. This is because of the effect of outliers.
Let’s take the above example and change some values and observe
Heights (in cm) = {180, 167, 154, 142, 181, 145, 143, 145, 167, 145}
According to observations,
We can see a significant change in mean whereas median does not have any changes.
This is because the calculation of mean incorporates all values in the data. If you change any value the mean changes.
Unlike the mean, median values does not depend on all the values in the data set,
Consequently, when some of the values are not extreme, the effect on median is smaller. of course, with other types of changes the median can change.
Hence, sometimes we must not use mean because it is particularly susceptible to extreme values or outliers in the data.